Bank Account Data
Make better decisions, faster
Securely access your user’s bank account information for better lending, accounting, verification, and financial management.
Over 900 businesses use Bank Account Data to deliver exceptional user experiences
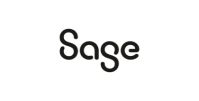
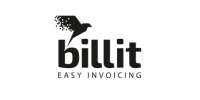
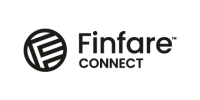
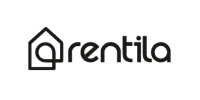
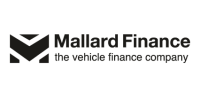
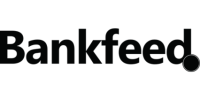
What you get

Account info and balance
Including account holder name, a list of their accounts with bank details and IBAN, as well as balance information.

Transaction history
Including the date, merchant name, transaction description, amount, and more.

Access 2,500+ banks
Connect personal, business and corporate bank accounts of 2,500+ banks across the UK and Europe.

Secure and regulated
No screen scraping or hacky workarounds. GoCardless is a licensed AISP, GDPR compliant, and ISO 27001 certified.
Bank Account Data Pricing
Free
Get access to bank account data without any financial commitment and make the most of 50 free connections per month.
£/€0
Free to use up to included monthly limit
Up to 50 free connections per month
Fetch data multiple times per day
Pay as you go
Gain full access to bank account data with unlimited connections, a customisable user interface and enhanced support, you can get to market quickly.
£/€100 per month
Plus £/€0.25 monthly price per connection
Unlimited connections
Fetch data multiple times per day
Customisable end user interface
Enhanced support
Company account verification
Custom pricing
If you have a large number of end users connecting their banks, please get in touch with our team for a custom pricing proposal.
Get custom quote
Based on expected number of connections
Unlimited connections
Fetch data multiple times per day
Customisable end user interface
Enhanced support
Company account verification
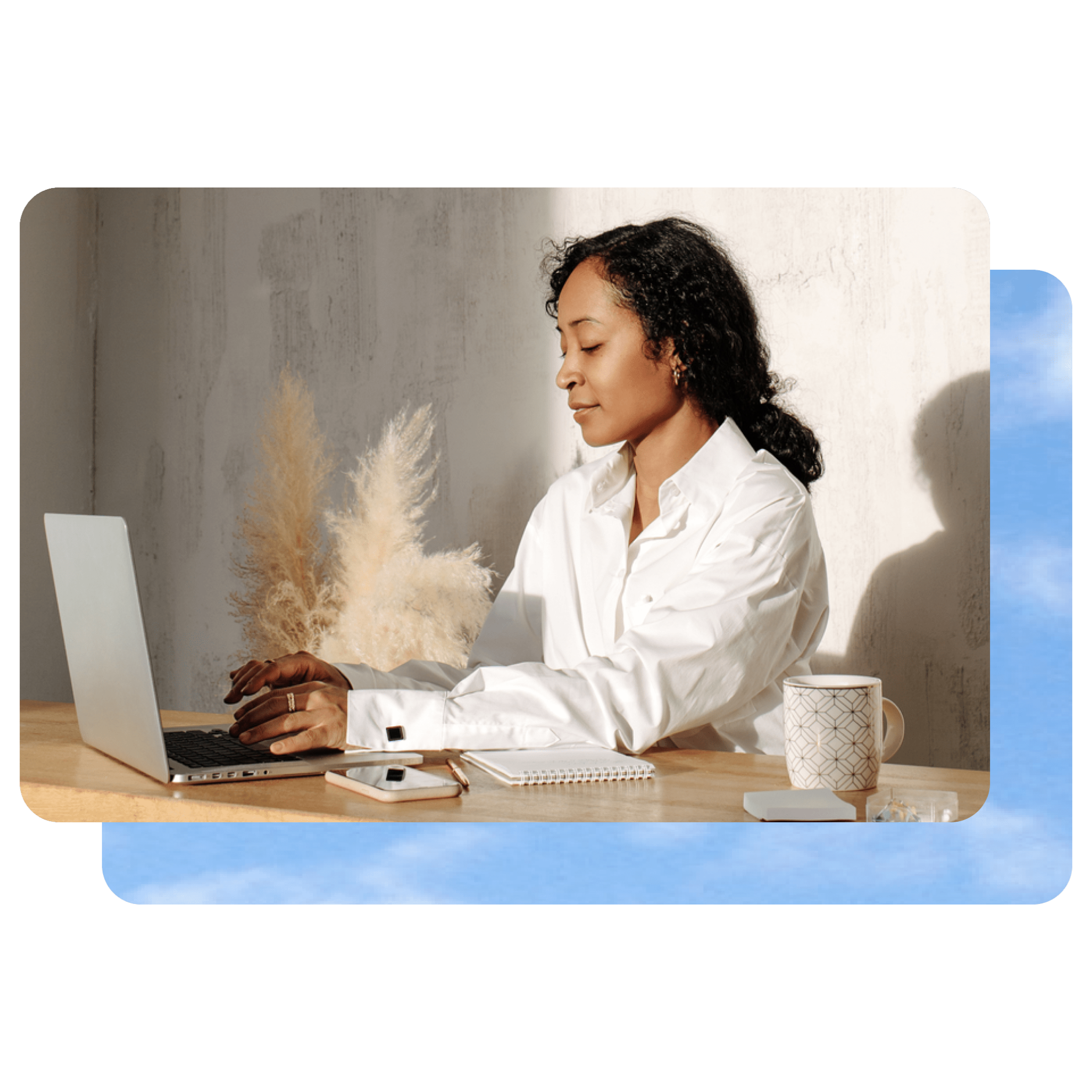
Use Bank Account Data for
How it works
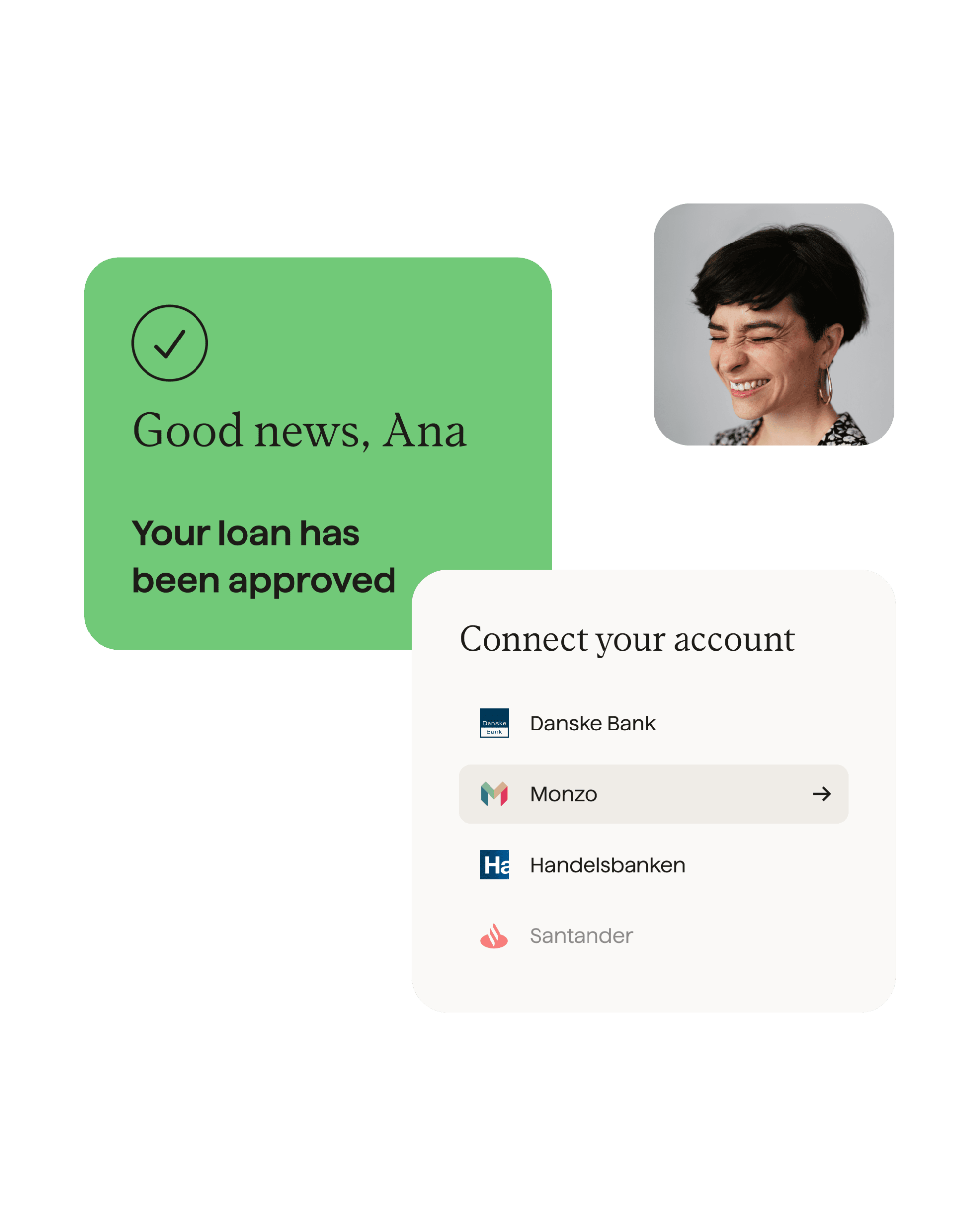
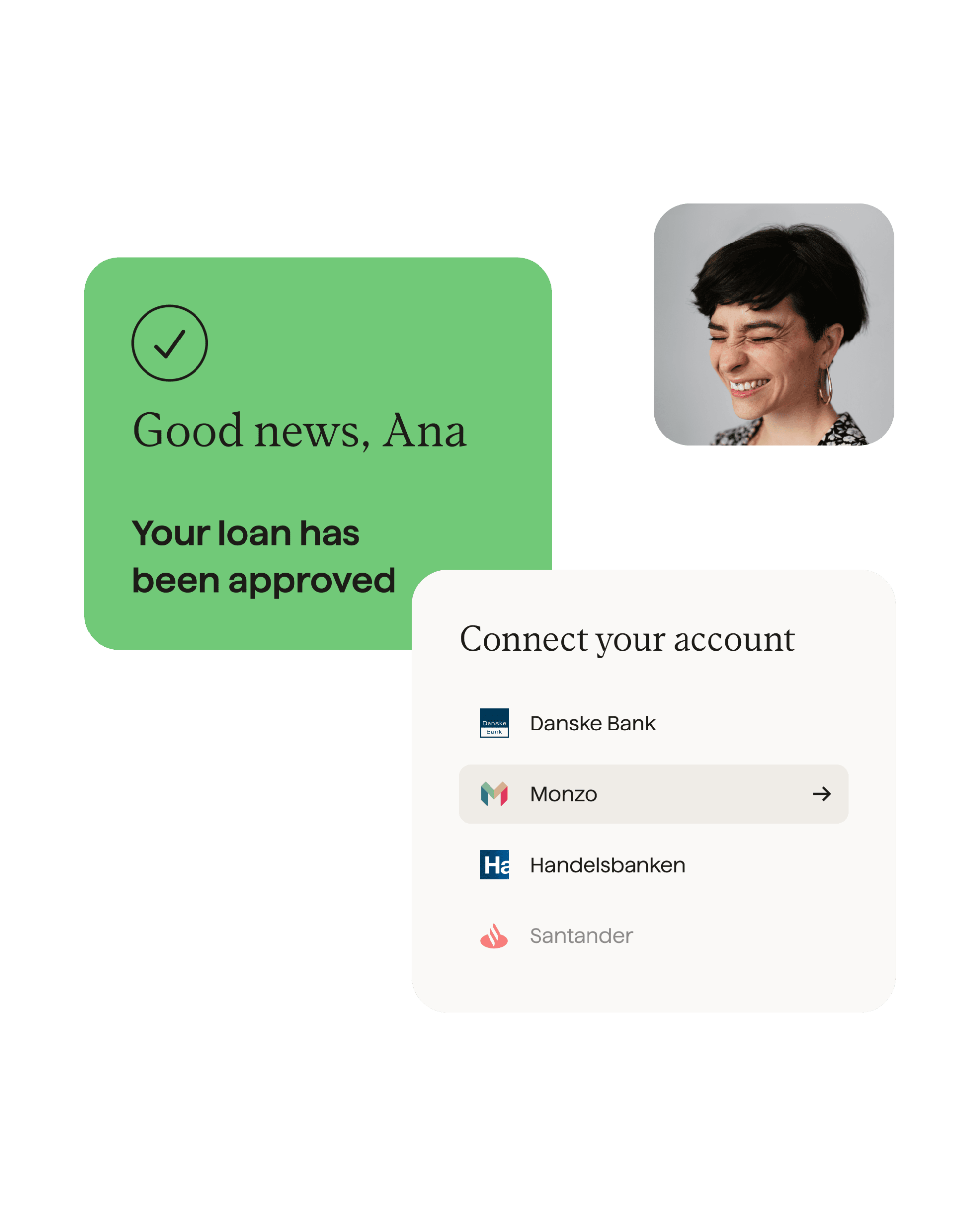
What sets us apart
Implement in hours, not weeks
Straightforward API implementation with a digestible quickstart guide to get you to market quickly.
Connect to more bank accounts
More than 2,500 connected banks in 31 countries across the UK and Europe.
No financial commitment to get started
Fast and easy setup with a free plan or pay-as-you go flexibility.
Responsive and personalised technical support
Top ranked (9.9 on G2) one-to-one customer support when, and where you need it.
GoCardless Bank Account Data provides fast implementation time
Fast and seamless integration using our quick and easy quickstart guide for GoCardless Bank Account Data API.
GoCardless Bank Account Data is delivering functionality that customers both need and expect for their business operations. It significantly simplifies and streamlines the reconciliation process, making it more efficient and user-friendly for our customers
Helen Pearson, Director of Product Management, Sage Network Banking and Payments Services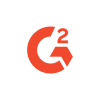
FAQs
Quick links
Ready to get started?
Create an experience your users will love. Power your software solution with GoCardless Bank Account Data.

